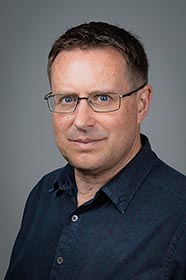
Head of Statistics
Director of Studies for 400-level and Honours Statistics
Director of Studies for postgraduate Statistics: Masters and PhD
Office: Science III, room 233
Tel +64 3 479 7605
Email martin.hazelton@otago.ac.nz
About
Martin Hazelton is a statistician with wide-ranging research interests (see below). He has worked previously at the University of Oxford, University College London, the University of Western Australia, and Massey University. Martin is editor-in-chief of the Australian and New Zealand Journal of Statistics.
Teaching responsibilities
teaching responsibilities include:
- STAT 110 Statistical Methods
- STAT 115 Introduction to Biostatistics
- STAT 310 Statistical Modelling
- STAT 405 Probability and Random Processes
Research Interests
I have a variety of research interests. These include:
Smoothing Methods
I have long been interested in kernel smoothing problems, and in particular spatially adaptive methods for multivariate data. Other areas of interest include kernel deconvolution problems and constrained spline smoothing.
Biostatistics and Applied Statistics
I have a keen interest in the development and application of statistical methods in medicine, particularly epidemiology and opthalmology.
Spatial Statistics
Through my interests in smoothing, networks, and geographical epidemiology, I have an evolving interest in spatial statistics.
I am Associate Investigator on a New Zealand Royal Society Marsden Fund grant entitled "A new generation of statistical models for spatial point process data" for 2020-2020. The project is led by my former PhD student Tilman Davies, and is in collaboration with Adrian Baddeley (Curtin University, Australia).
Statistical Modelling and Inference in Transportation Science
Transportation science generates a huge range of fascinating problems. I'm currently focused on network tomography (in essence, statistical methods for learning about high dimensional properties of network traffic flows based on lower dimensional observations), and modelling and inference for day-to-day dynamic traffic networks.
Statistical Linear Inverse Problems and Z-Polytope Sampling
Statistical linear inverse problems are characterized by the linear system y = Ax where y is a vector of observed data and x is the variable of principal interest. The configuration matrix A typically has (many) more columns than rows, so that the linear system is under-determined. A classic example is network tomography, where we want to know about traffic flows x on paths through the network but we observe only traffic counts y at various network locations. Other examples with the same structure include (re)sampling entries of a contingency table conditional on various marginal totals, counts of individual animals in capture-recapture experiments in ecology where misidentification may occur (so that the true counts x differ from the observed counts y), and assessment of items for biosecurity risk under stratified sampling.
When the data are counts, the observations y constrain the variables of interest x to lie in a Z-polytope - that is, the grid of integer valued coordinates (yellow dots in the figure to the right) within a multidimensional polyhedron. Practical methods of statistical inference (like MCMC) require that we sample vectors x lying in this Z-polytope. This is typically done using a random walk. The problem then is to construct a random walk that traverses the Z-polytope efficiently and yet always remains within its bounds. It turns out that this is a hard problem!
I have recently been awarded a Marsden Fund grant as lead researcher on the project "Inference for statistical linear inverse problems: theory and practice" (2021-2024), working with Rachel Fewster, Jesse Goodman (both University of Auckland) and Andrew Robinson (University of Melbourne). This research will examine methods of inference based on Z-polytope sampling, and also likelihood-based approaches using saddlepoint approximations (an area in which my Auckland-based collaborators are expert).
Other Research Topics
In addition to these medical areas, I have a general interest in the application of statistical methods. Indeed, one of the great things about working in statistics is that I've had the opportunity to look at a diverse range of intriguing problems from a wide variety of areas, from archaeology, to finance, to zoology.